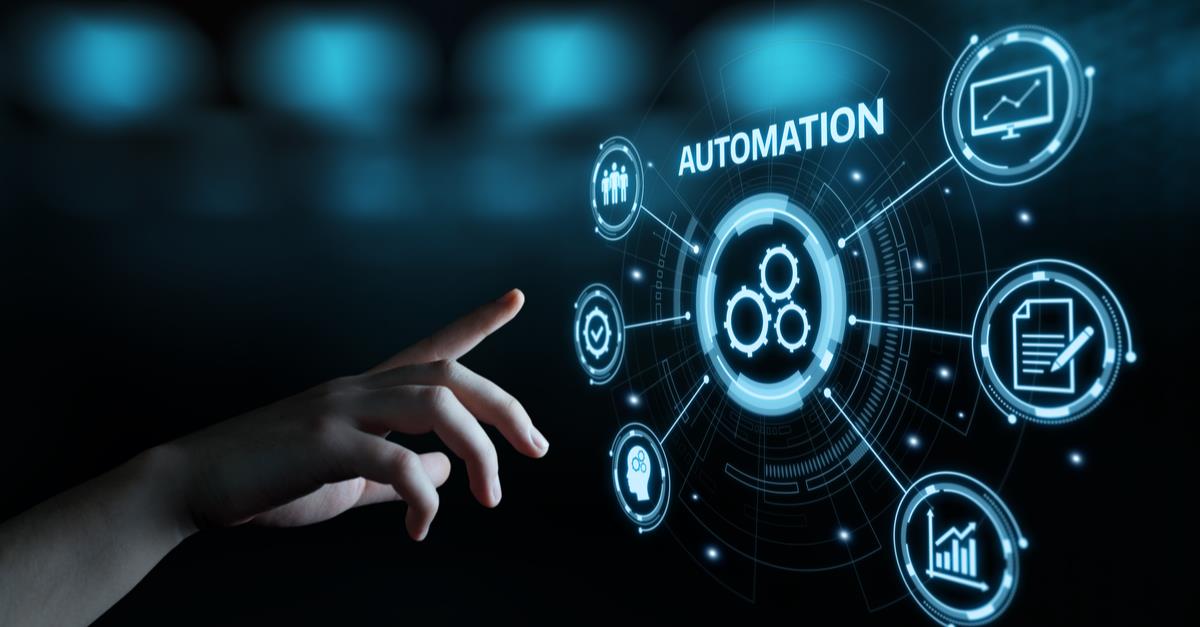
In the ever-evolving landscape of cybersecurity, the integration of artificial intelligence (AI) has become a paramount concern. This article delves into the ten key aspects where AI intersects with cybersecurity, providing an in-depth analysis of:
- Threat detection
- Fraud prevention
- Data privacy
- Network security
- Risk management
- Predictive analytics
- Identity verification
- Encryption
- Cyber defense
By examining these critical areas, organizations can enhance their understanding of AI's role in safeguarding against emerging threats and fortifying their digital ecosystems.
Threat Detection
Threat detection is a critical component of cybersecurity, as it involves identifying and mitigating potential risks and vulnerabilities in computer systems and networks.
In order to effectively detect threats, various techniques are employed, including anomaly detection and behavioral analysis.
Anomaly detection focuses on identifying deviations from normal patterns of behavior within a system. By establishing a baseline of normal behavior, any deviations can be flagged as potential threats. This technique is particularly useful in detecting unknown or zero-day attacks that have not yet been identified.
Behavioral analysis, on the other hand, involves monitoring and analyzing the actions and interactions of users and devices within a network. By establishing typical behavior patterns, any deviations or suspicious activities can be detected and investigated.
These proactive measures help organizations identify and respond to potential threats before they can cause significant damage to their systems and networks.
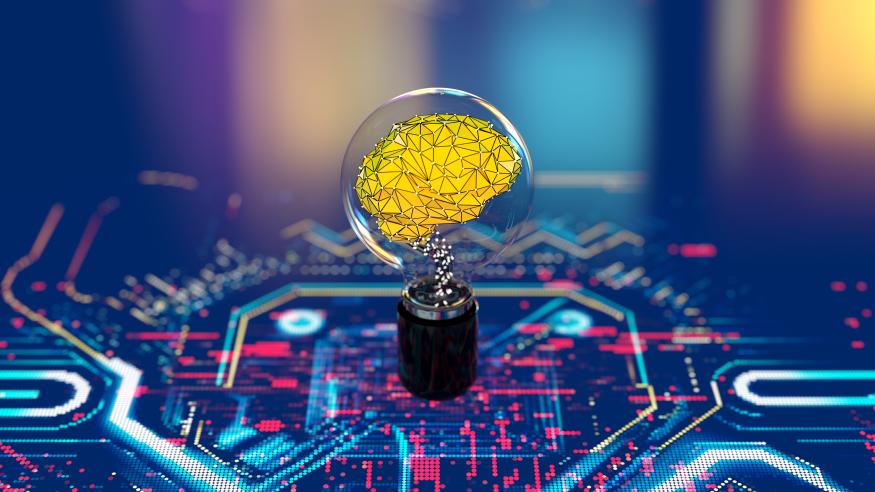
Fraud Prevention
In the realm of cybersecurity, an essential aspect that builds upon threat detection is fraud prevention. As technology advances, cybercriminals are becoming more sophisticated in their tactics, making it crucial for organizations to implement robust fraud prevention measures.
One such measure is behavior analysis, which involves analyzing users' actions and patterns to identify any suspicious behavior that may indicate fraudulent activity. Machine learning techniques play a vital role in this process by enabling systems to learn and adapt to new fraud patterns in real-time.
By leveraging machine learning algorithms, organizations can detect and prevent fraud more effectively, ensuring the security and trust of their systems and users. In the fight against cybercrime, fraud prevention is a critical component that safeguards the freedom and privacy of individuals and organizations alike.
Efficiently detect and prevent fraudulent activities.
Proactively identify suspicious behavior.
Enhance system security and user trust.
Real-time adaptation to emerging fraud patterns.
Safeguard freedom and privacy.
Data Privacy
Data privacy is a critical concern in the realm of AI and cybersecurity.
To address this issue, encryption methods play a vital role in protecting sensitive data.
However, it is essential to strike a balance between data protection and the need for data accessibility and usability.
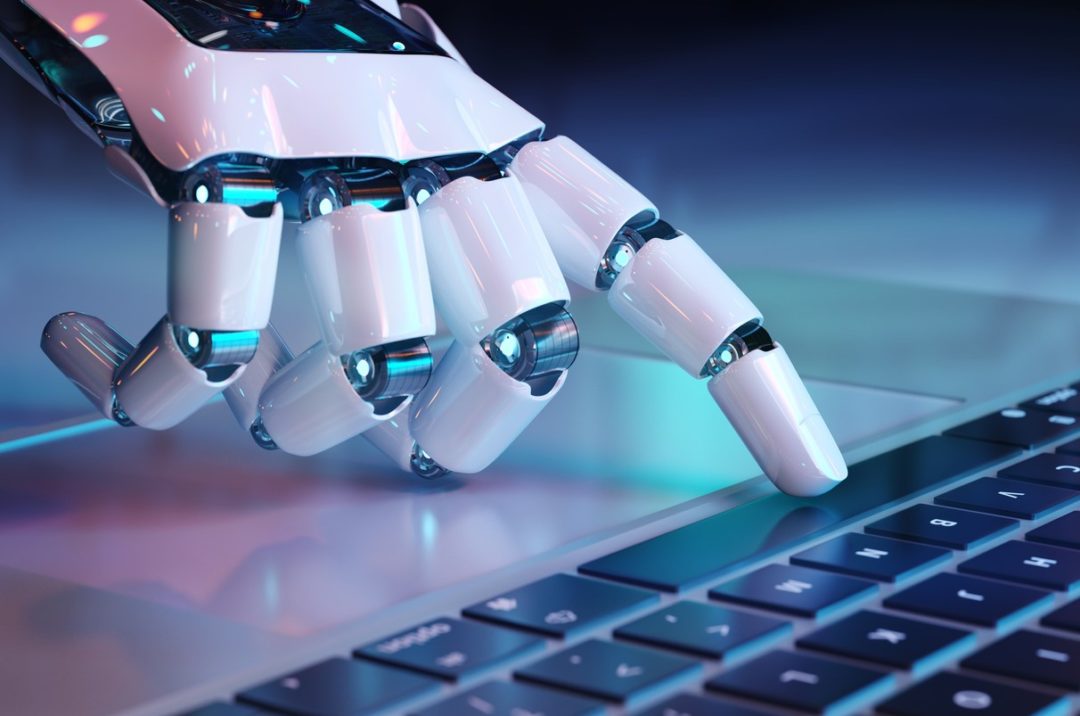
AI and Encryption Methods
With regards to AI and cybersecurity, one crucial aspect to consider is the integration of encryption methods to ensure data privacy.
In the age of AI and quantum computing, traditional encryption algorithms are becoming vulnerable, necessitating the development of new techniques. Machine learning is playing a pivotal role in enhancing encryption methods by enabling the creation of more robust and adaptive algorithms.
Here are five key points to understand about AI and encryption methods:
- AI-powered encryption algorithms can dynamically adapt to evolving threats, providing enhanced security.
- Machine learning algorithms can analyze large datasets to identify patterns and anomalies, improving encryption key management.
- Quantum computing poses a significant threat to traditional encryption methods, driving the need for quantum-resistant encryption algorithms.
- AI can help automate the encryption process, reducing human error and ensuring consistent implementation.
- AI-powered encryption can provide end-to-end data protection, safeguarding sensitive information from unauthorized access.
Balancing Data Protection
To ensure comprehensive cybersecurity, it is essential to strike a balance between preserving data privacy and implementing robust protection measures.
Data breach prevention and privacy regulations compliance are two key aspects of this balancing act.
Organizations must prioritize data protection by implementing strong security measures to safeguard sensitive information from unauthorized access, theft, or misuse. This includes employing encryption techniques, access controls, and secure communication channels.
At the same time, organizations must also comply with privacy regulations to respect individuals' right to control their personal information. This involves obtaining explicit consent for data collection and processing, providing transparent privacy policies, and implementing mechanisms for individuals to exercise their data rights.
Network Security
Network security plays a crucial role in safeguarding organizations from emerging threats in the digital landscape. With the advancements in AI, it has become a key component in network defense, allowing for real-time threat detection and response.
However, as threats continue to evolve, it is essential to explore and implement innovative solutions that can proactively mitigate these risks and ensure the integrity and confidentiality of network data.
AI for Network Defense
The implementation of AI technology in network defense is crucial for safeguarding against cyber threats. AI-powered network monitoring tools can analyze vast amounts of data in real-time, enabling organizations to detect and respond to potential security breaches more effectively.
Anomaly detection, one of the key capabilities of AI in network defense, helps to identify deviations from normal network behavior, allowing for the early detection of malicious activities. With AI, network defenders can leverage advanced algorithms and machine learning to continuously learn and adapt to evolving threats, enhancing the overall security posture.
This empowers organizations to proactively protect their networks, minimize the impact of cyber attacks, and ensure the freedom to operate securely in the digital landscape.
- Enhanced threat detection capabilities
- Real-time monitoring and response
- Improved accuracy in identifying anomalies
- Continuous learning and adaptation
- Strengthened overall network security
Emerging Threats and Solutions
Emerging threats in network security pose significant challenges that require proactive measures to mitigate their potential impact. With the rapid advancement of emerging technologies, such as the Internet of Things (IoT) and cloud computing, the attack surface for cybercriminals continues to expand. Traditional security measures are often ill-equipped to deal with these new threats, necessitating the integration of emerging technologies and machine learning applications.
One of the major emerging threats in network security is the proliferation of sophisticated malware and ransomware attacks. These attacks can infiltrate networks and systems, causing significant disruption and financial losses. To combat these threats, organizations are increasingly turning to machine learning applications that can analyze vast amounts of data in real-time to detect and respond to anomalies and suspicious activities.
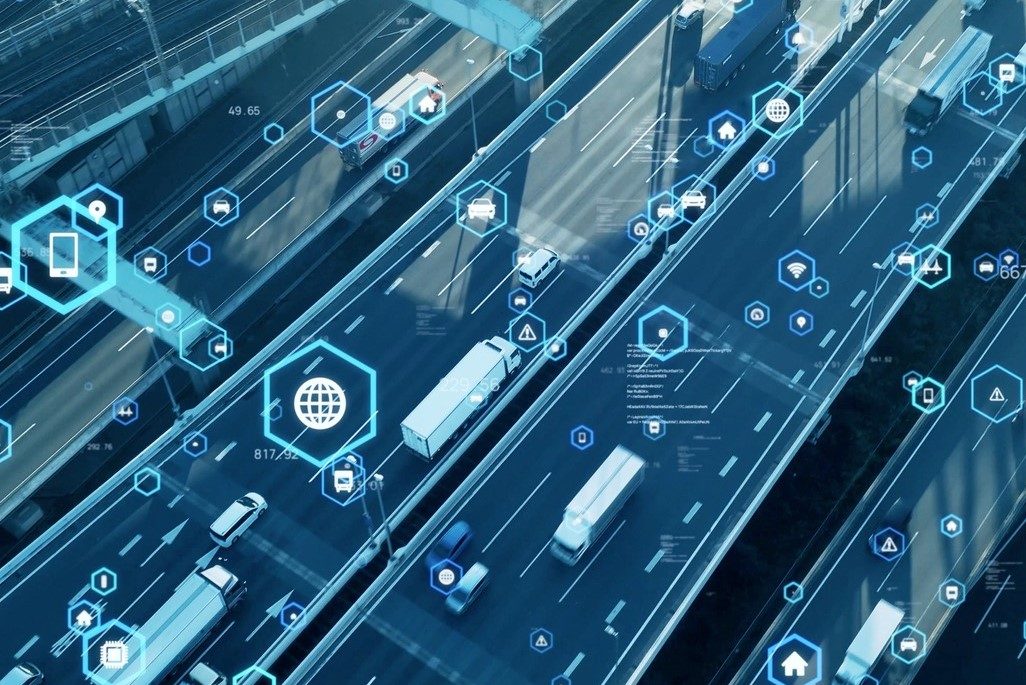
Additionally, the rise of insider threats and social engineering attacks has further highlighted the need for advanced network security solutions. Machine learning algorithms can analyze user behavior and detect patterns that may indicate malicious intent, enabling organizations to proactively address potential threats before they cause significant damage.
Risk Management
To effectively protect against cyber threats, organizations must prioritize risk management in the context of AI and cybersecurity. Risk assessment and vulnerability management are crucial components of this process. Here are some key aspects to consider:
- Continuous monitoring: Organizations should implement real-time monitoring systems to detect and respond to potential risks promptly.
- Threat intelligence: Staying informed about the latest cyber threats and attack techniques allows organizations to proactively address vulnerabilities.
- Incident response planning: Developing a well-defined incident response plan enables organizations to mitigate the impact of cyber attacks effectively.
- Employee training and awareness: Educating employees about cybersecurity best practices and raising awareness about potential risks can significantly reduce the likelihood of successful attacks.
- Regular audits and assessments: Conducting periodic audits and assessments help identify weaknesses in existing security measures and ensure compliance with industry standards and regulations.
Incident Response
Incident response plays a crucial role in effectively addressing and mitigating cyber attacks by promptly detecting and responding to security incidents. In today's complex threat landscape, organizations need to have a robust incident response plan in place to minimize the impact of cyber threats.
Incident response involves a series of steps, including preparation, detection, analysis, containment, eradication, and recovery. It requires a combination of technical expertise, effective communication, and the utilization of threat intelligence.
Threat intelligence provides valuable insights into the latest attack vectors, tactics, and vulnerabilities, enabling organizations to better understand and respond to security incidents. By leveraging threat intelligence, incident response teams can stay proactive in identifying potential threats, developing effective countermeasures, and minimizing the impact of cyber attacks on their systems and data.
Predictive Analytics
Utilizing predictive analytics is a crucial aspect of implementing effective AI-driven cybersecurity measures. Predictive modeling and machine learning algorithms play a key role in analyzing vast amounts of data to identify potential cyber threats before they occur. This proactive approach allows organizations to stay one step ahead of cybercriminals and take preventive measures to safeguard their systems and data.
Here are five reasons why predictive analytics is essential in cybersecurity:
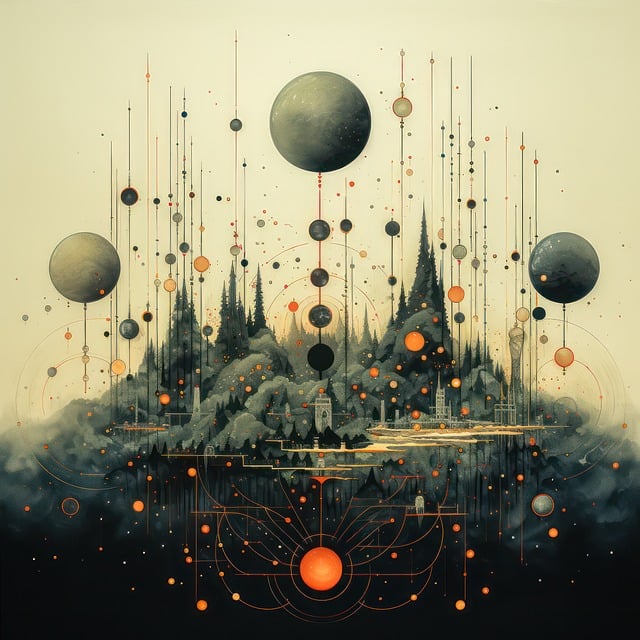
- Early detection of cyber threats, enabling timely response and mitigation.
- Identification of patterns and trends that indicate potential attacks.
- Enhanced accuracy in predicting future cyber threats.
- Reduction in false positives, minimizing unnecessary alerts and distractions.
- Improved decision-making by providing actionable intelligence to security teams.
Identity Verification
Identity verification is a critical aspect of cybersecurity, ensuring that individuals accessing systems and data are who they claim to be. Biometric authentication methods, such as fingerprint or facial recognition, provide a high level of security by leveraging unique physical characteristics.
Deepfake detection technology plays a crucial role in preventing fraudulent activities, detecting manipulated or synthetic identities.
Real-time identity verification solutions enable organizations to verify identities in a seamless and efficient manner, enhancing security and reducing the risk of unauthorized access.
Biometric Authentication Methods
Biometric authentication methods play a crucial role in verifying individual identities in the field of AI and cybersecurity. These methods utilize unique biological traits, such as fingerprints, iris patterns, or facial features, to accurately identify and authenticate individuals.
However, despite their effectiveness, the implementation of biometric authentication faces several challenges. These challenges include:
- Privacy concerns: The collection and storage of biometric data raise concerns about individuals' privacy and the potential for misuse or unauthorized access.
- Accuracy and reliability: Biometric systems must be accurate and reliable, ensuring that false positives or false negatives are minimized.
- Scalability: Implementing biometric authentication on a large scale, such as in an organization or at a national level, requires robust infrastructure and resources.
- Interoperability: Different biometric systems may use different algorithms or standards, making it challenging to integrate them seamlessly.
- Spoofing and tampering: Biometric systems need to be resilient against spoofing attacks, where adversaries attempt to impersonate someone else's biometric traits.
Deepfake Detection Technology
Continuing the discussion from the previous subtopic, deepfake detection technology plays a crucial role in verifying individual identities in the field of AI and cybersecurity.
As deepfake technology advances, so do the challenges in detecting and preventing its malicious use. Deepfake detection algorithms have become indispensable tools in identifying manipulated or synthetic media content. These algorithms analyze various features such as facial expressions, eye movements, and voice patterns to distinguish between genuine and deepfake videos or audios.
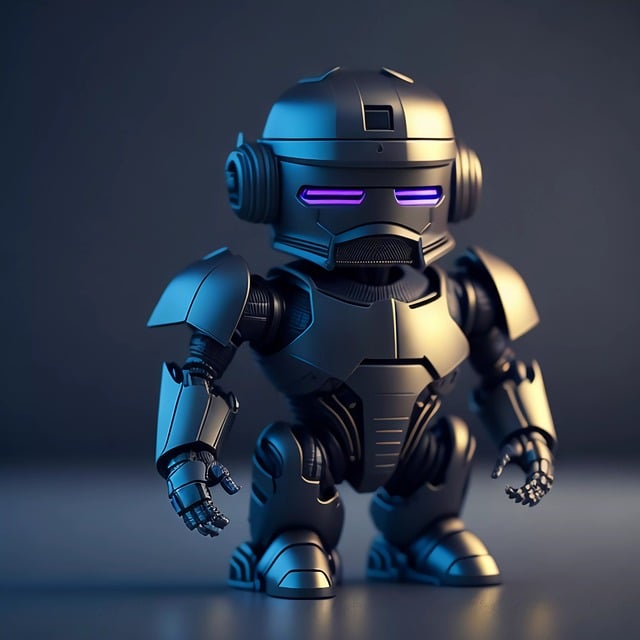
However, deepfake detection still faces significant challenges. Adversarial attacks, where deepfake creation methods are specifically designed to deceive detection algorithms, pose a major obstacle. Additionally, the rapid evolution of deepfake techniques requires continuous updates and improvements to detection algorithms to remain effective in identifying and combatting this emerging threat to identity verification and cybersecurity.
Real-Time Identity Verification
As advancements in deepfake detection technology continue to address challenges in verifying individual identities, real-time identity verification emerges as a critical aspect of AI and cybersecurity.
With the rise of cyber threats and the increasing sophistication of identity fraud, it is essential to have robust systems that can accurately and efficiently verify the identity of individuals in real-time.
Real-time identity verification utilizes a combination of behavior analysis and facial recognition to ensure that the person claiming an identity is indeed who they say they are.
This advanced technology not only enhances security measures but also streamlines processes, reducing the risk of identity theft and providing individuals with the freedom to transact securely and confidently.
Encryption
With regards to AI and cybersecurity, encryption plays a critical role in safeguarding sensitive information.
Data encryption is the process of converting plaintext into ciphertext, making it unreadable to unauthorized individuals. It ensures the confidentiality and integrity of data by using complex algorithms and keys.
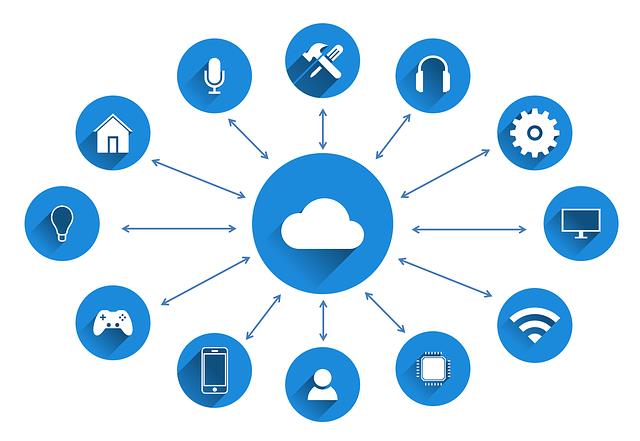
Encryption is essential for secure communication, preventing eavesdropping and unauthorized access to information. It acts as a protective shield, especially in the era of increasing cyber threats and data breaches.
Encryption technologies like symmetric encryption, asymmetric encryption, and hashing algorithms are widely used to secure data in transit and at rest.
Implementing strong encryption measures is crucial to maintaining privacy and ensuring the security of sensitive information in the digital age.
Cyber Defense
The foundation of effective cybersecurity lies in a robust cyber defense strategy. To combat the ever-evolving cyber threats, organizations need to employ advanced techniques and technologies.
Here are five crucial elements that form the backbone of a strong cyber defense:
- Cyber Threat Intelligence: Gathering and analyzing information about potential threats enables proactive identification and mitigation of risks.
- Security Operations: Implementing a well-defined set of processes and procedures ensures efficient handling of security incidents.
- Continuous Monitoring: Regularly monitoring networks and systems allows for the timely detection and response to any suspicious activities or breaches.
- Incident Response: Having a well-prepared incident response plan minimizes the impact of security incidents and facilitates swift recovery.
- Threat Hunting: Proactively searching for signs of malicious activity helps in identifying and neutralizing potential threats before they cause harm.
Frequently Asked Questions
How Can AI Help in Identifying and Preventing Emerging Cyber Threats?
AI powered threat intelligence, leveraging machine learning for real time threat detection, can help in identifying and preventing emerging cyber threats. By analyzing vast amounts of data and patterns, AI can detect anomalies and mitigate potential risks before they cause significant damage.
What Are the Major Challenges Faced in Implementing AI for Fraud Prevention in Cybersecurity?
Implementing AI for fraud prevention in cybersecurity presents challenges such as data quality, limited labeled data sets, and adversarial attacks. Strategies to address these challenges include developing robust models, improving data collection, and implementing proactive defense mechanisms.
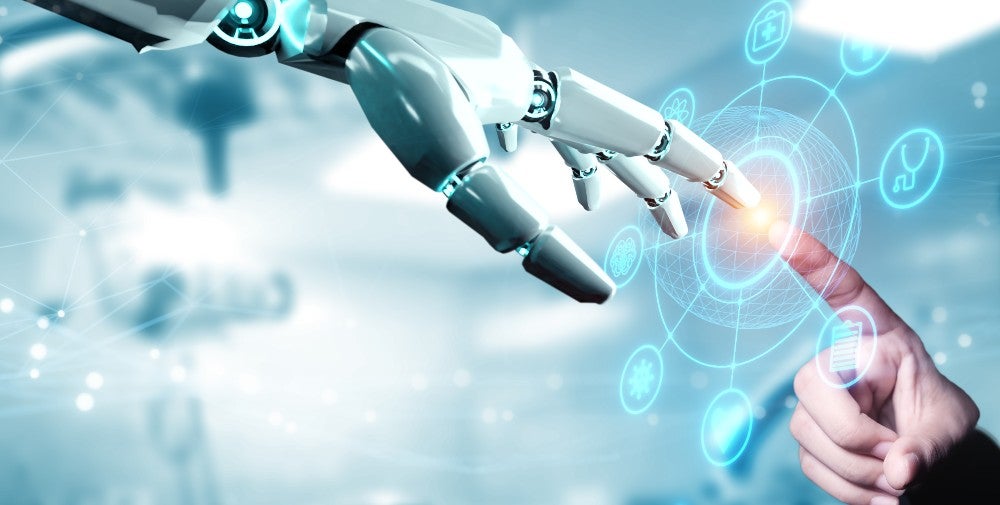
How Does Data Privacy Regulation Impact the Use of AI in Cybersecurity?
Data privacy regulation impacts the use of AI in cybersecurity by imposing strict guidelines on the collection, storage, and processing of personal data. It addresses the ethical implications of AI, ensuring the protection of individuals' privacy and promoting transparency and accountability in cybersecurity practices.
What Are the Key Techniques Used in Ai-Based Network Security Solutions?
Behavior analysis and anomaly detection are key techniques used in AI-based network security solutions. These techniques enable the identification of unusual patterns and behaviors in network traffic, helping to detect and prevent cyber threats.
How Can AI Be Utilized in Incident Response to Minimize the Impact of Cyberattacks?
Incident response automation leveraging AI can minimize the impact of cyberattacks. Machine learning algorithms can quickly analyze vast amounts of data, detect anomalies, and respond to threats in real-time, enhancing the efficiency and effectiveness of incident response teams.